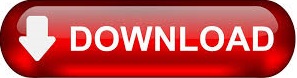

Here we look at pairs of cells from R1 and R2: the ith cell in R1 is paired with the ith cell in R2ĬountPairs(R1, R2, TRUE) = the number of pairs for which neither cell in the pair is emptyĬountPairs(R1, R2, FALSE) = the number of pairs for which neither cell in the pair is empty or non-numeric There is also the related Real Statistics function CountPairs(R1, R2, blank) where blank = TRUE (default) or FALSE. In addition, there is the function CountFullRows(R1, blank) where blank = TRUE (default) or FALSE.ĬountFullRows(R1, TRUE) = the number of rows in range R1 which don’t have any empty cellsĬountFullRows(R1, FALSE) = the number of rows in range R1 which don’t have any non-numeric cells The following additional function is useful with dynamic arrays (see Dynamic Array Formulas).ĭELROWS(R1, head, blank): outputs an array with the data in R1 omitting any row that has one or more blank elements if blank = TRUE or one or more non-numeric elements if blank = FALSE (default) if head = TRUE, then the first row is always included in the output otherwise (default), the first row is treated like any other row. See Data Conversion and Reformatting for an example of the use of these functions. This argument is optional and defaults to the error value #N/A. The string s is used as a filler in case the output range has more cells/rows than needed. Missing data can be removed by using the following functions found in the Real Statistics Resource Pack.ĭELBLANK(R1, s) – fills the highlighted range with the data in range R1 (by columns) omitting any empty cellsĭELNonNum(R1, s) – fills the highlighted range with the data in range R1 (by columns) omitting any non-numeric cellsĭELROWBLANK(R1, head, s) – fills the highlighted range with the data in range R1 omitting any row which has one or more empty cells if head is TRUE then the first row of R1 (presumably containing column headings) is always copied (even if it contains an empty cell) this argument is optional and defaults to head = FALSE.ĭELROWNonNum(R1, head, s) – fills the highlighted range with the data in range R1 omitting any row which has one or more non-numeric cells if head is TRUE then the first row of R1 (presumably containing column headings) is always copied (even if it contains a non-numeric cell) this argument is optional and defaults to head = FALSE. by adding a “no response” for missing data) and once with these samples dropped. In this case, either another remedy should be employed or the analysis should be run twice: once with samples with missing data retained (e.g. If the frequency of the responses to question 7 changes significantly when samples that are missing responses to question 5 are dropped, then the missing data is not random, and so dropping samples can bias the results of the analysis. suppose a lot of people didn’t answer question 5 but everyone answered question 7. Of particular importance is the randomness of the missing data. questions) that measure similar aspects of the characteristics being studied.
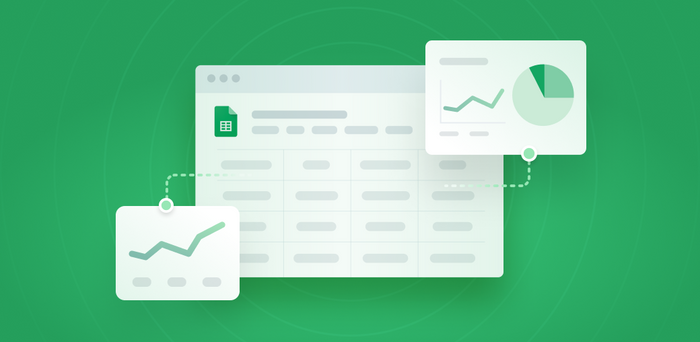
a particular question in the case of a questionnaire or survey) that has a high incidence of missing data, especially if there are other variables (e.g. Delete the samples with any missing data elements.In general, there are the following types of remedies for missing data: These are characteristics that might be quite relevant to the analysis. people filling out a long questionnaire may give up at some point and not answer any further questions, or they may be offended or embarrassed by a particular question and choose not to answer it. if a questionnaire with 5 questions is randomly missing 10% of the data, then on average about 41% of the sample will have at least one question missing.Īlso, it is often the case that the missing data is not randomly distributed. This problem is bigger than might first be evident. In this case, additional sample data elements may need to be collected. This is particularly relevant when the reduced sample size is too small to obtain significant results in the analysis. One problem with this approach is that the sample size will be reduced.
DATA ANALYSIS EXCEL NOT SHOWING HOW TO
In Identifying Outliers and Missing Data we show how to identify missing data using a data analysis tool provided in the Real Statistics Resource Pack.Ī simple approach for dealing with missing data is to throw out all the data for any sample missing one or more data elements. For example, in conducting a survey with ten questions, perhaps some of the people who take the survey don’t answer all ten questions. One problem faced when collecting data is that some of the data may be missing.
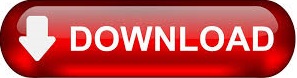